Composite AI provides an extra dimension for businesses looking for growth, compliance and profitability
Single AI technologies are useful, but lack the added context, insight and edge that a combination of AI techniques can provide
While the first wave of digital transformation centered on the digitalization of business processes and offerings, including products, services, and experiences; artificial intelligence (AI) is driving the second wave, enabling organizations to take a more holistic approach to harnessing and analyzing data in order to further improve decision-making, staff productivity, and operational efficiency.
Enterprises have found AI techniques such as machine learning particularly valuable in deriving insights through data analytics, and are now going beyond to even more effectively harness their data by using machine learning in conjunction with other AI techniques and advanced technologies. This is essentially the emerging theme of Composite AI, which Gartner recently recognized under the ‘Innovation Trigger’ of its Hype Cycle for Artificial Intelligence, 2021. In the previous year, Gartner’s Hype Cycle of Emerging Technologies, 2020 report identified Composite AI as one of the top 30 technologies with the highest degree of predicted impact on business and society in the next several years. This was after evaluating 1,700 technologies.
Composite AI combines multiple AI techniques
Composite AI is a breakthrough approach that combines multiple AI techniques to efficiently solve a wider range of business problems by more deeply interpreting data. These AI techniques may include natural language processing, machine learning, and deep learning; and other advanced technologies such as knowledge graphs.
Knowledge graph technology contextualizes massive amounts of data in a way similar to the human mind, while relying on data management processes that involve a number of AI techniques to thoroughly interpret the data. As a live information asset, the technology builds a comprehensive representation of entities and all the relationships between them using conceptual maps. These graphs can be further enriched with structured and unstructured data from a variety of internal and external sources, such as social media and global news, to ensure that all relevant information is included. Knowledge graphs can also evolve over time to reflect the most up-to-date state of intelligence.
Natural language processing combines computational linguistics — rule-based modeling of human language — with other AI techniques such as machine learning and deep learning to analyze text written or words spoken by human beings. Thus, by converting human language into a machine-understandable format, natural language processing systems enable computers and humans to interact with each other.
Machine learning algorithms power self-learning models that learn how to make predictions based on historical sample data and automatically improve through experience. These algorithms are commonly used to classify information in order to understand the context. When combined with knowledge graph technology, machine learning algorithms can be used to continuously update the graph and create new knowledge about the objects and their relationships. Machine learning algorithms can also uncover non-obvious relationships between the entities then use that knowledge to categorize and organize the data.
While machine learning uses basic neural networks to solve problems involving structured data, deep learning algorithms leverage more complex neural networks to solve even more complicated problems involving either structured or unstructured data or both. When combined with multi-level semantic natural language processing, these AI technologies can break each article down to the sentence-level, resolving the nature of each entity and their respective actions.
All these technologies combine to help decision-makers gain insights of unprecedented granularity, depth, and precision by responding to queries with a representation of all relevant pieces of information in one consistent conceptual map. This overview of all data and relationships between them is particularly beneficial when making decisions within the realm of business challenges such as due diligence and compliance.
The democratization of AI has further fueled the widespread adoption of AI technologies, enabling users of all skill levels to develop intelligent business applications. This, in combination with Composite AI, has empowered business teams with augmented intelligence while minimizing the need for specialized data scientists and lengthy data integration projects. As a result, business teams are able to focus more on problems requiring higher levels of intellect and creativity.
Composite AI in action
Enterprises across various industries are already benefiting from the use of a Composite AI approach. In one such example, an insurance company uses multiple AI techniques to select the best coverage plan for a new customer.
Knowledge graph technology is first utilized to gather all relevant information about the prospective customer such as company overview, insurance history, relationships, and related news. The graph is further enriched with all relevant domain knowledge about the insurance plans available along with various criteria such as location, industry, and customer base.
The customer is analyzed to propose a path for the resolution of the case based on AI inference rules and graph analysis. Then, the system automatically initiates contact with a link to an AI-powered chatbot to negotiate an insurance proposal. The system also clarifies other relevant details with the customer to explore additional opportunities. If needed, the system will engage an account manager to work further with the customer. Finally, the system analyzes the outcome of the case to learn and automatically improve.
As organizations remain under constant pressure to maintain a competitive advantage in the face of the ever-growing threat of new players, AI will prevail as the key driver of digital transformation. Thus, to compete and thrive, organizations will have to adopt AI technologies while capitalizing on their vast amounts of historical data — an unfair advantage over newcomers. With Composite AI, organizations can go one step further by combining multiple AI techniques to maximize the strengths and minimize the weaknesses of each.
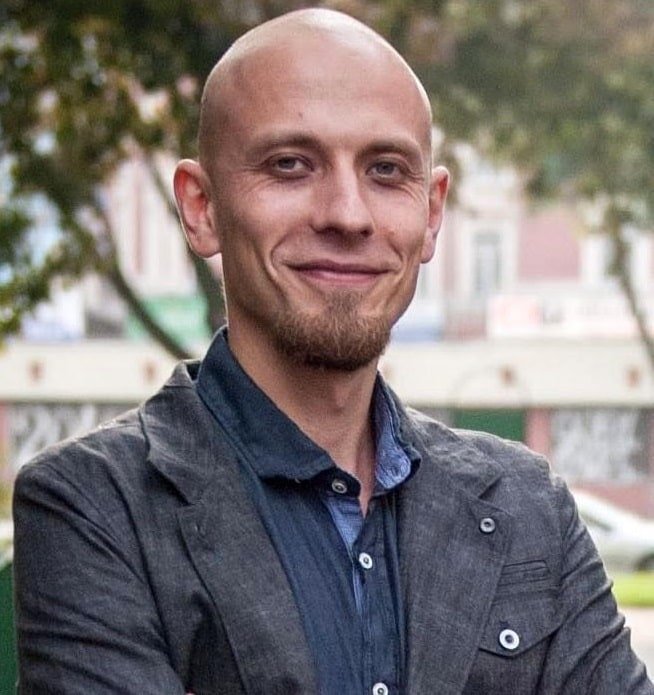
Szymon Klarman; Knowledge Architect at BlackSwan Technologies